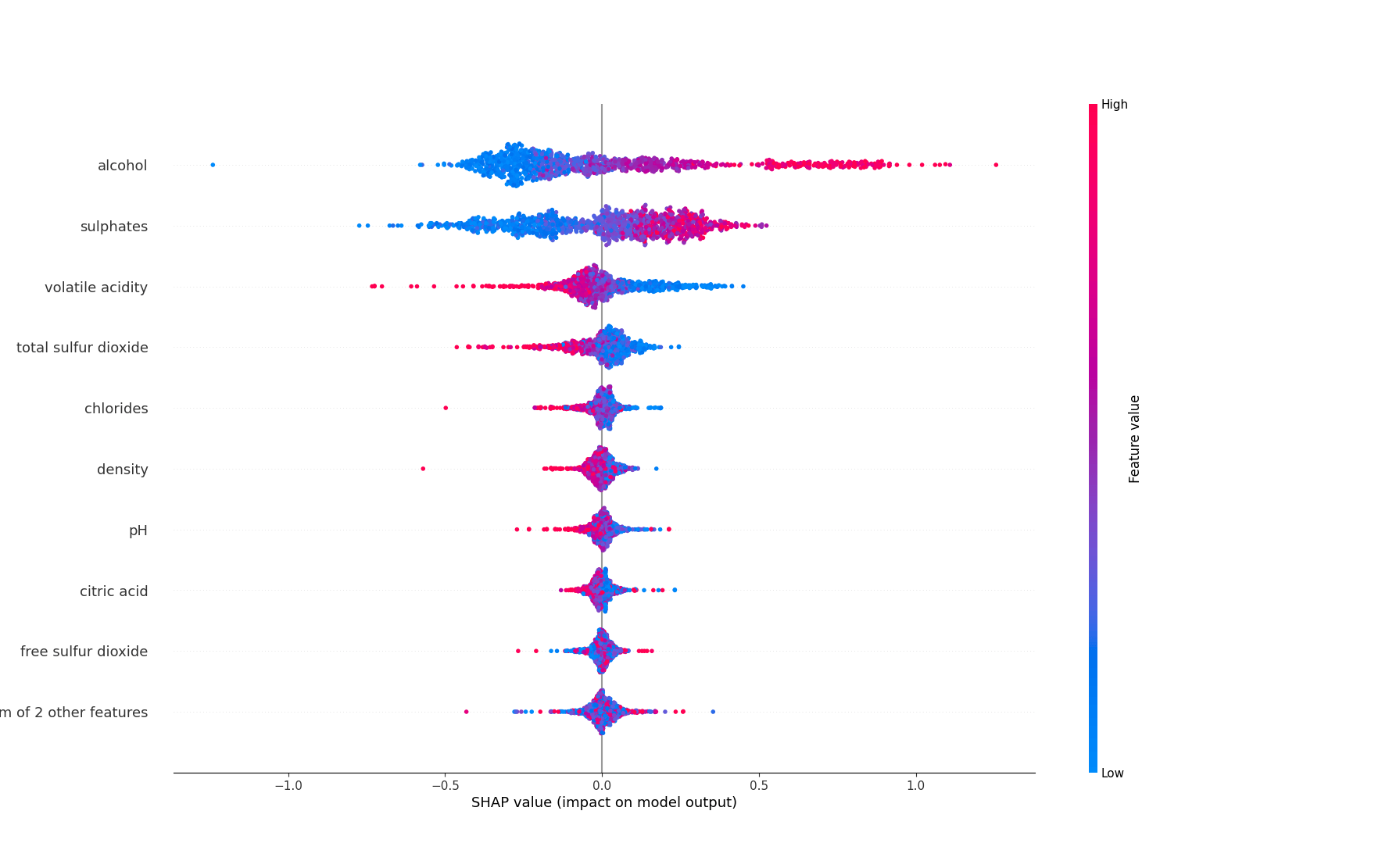
Calculando Importância de Features em Random Forests
Interpretar e identificar as features cruciais em modelos de machine learning pode ser um desafio e tanto, especialmente ao lidar com modelos black-box. Neste tutorial, vamos mergulhar fundo no entendimento da importância global e local das features em Random Forests. Exploraremos várias técnicas e ferramentas para analisar e interpretar essas importâncias, tornando nossos modelos mais transparentes e confiáveis. Para ilustrar as técnicas, utilizaremos o conjunto de dados “Red Wine Quality” do Repositório de Machine Learning da UCI....